In the fiercely competitive landscape of the National Hockey League (NHL), teams like the New York Rangers and the Carolina Hurricanes are turning to advanced technologies to gain an edge. At the forefront of this technological revolution are players like Igor Shesterkin of the Rangers and Andrei Svechnikov of the Hurricanes, whose performances are being dramatically enhanced by the integration of machine learning in their training and game analysis.
Revolutionizing Hockey Through Data
Machine learning’s capability to process vast datasets to extract actionable insights is transforming sports analytics. This technology is particularly influential in optimizing the performance of NHL stars such as goaltender Igor Shesterkin and forward Andrei Svechnikov.
Analyzing Goalkeeping Excellence: Igor Shesterkin
Igor Shesterkin’s prowess in the net for the New York Rangers can be dissected through AI-driven metrics such as save percentage, goals-against average, and reaction times. These models predict Shesterkin’s performance trends, enabling tailored training that addresses specific weaknesses identified by the data. For example, if analysis shows that Shesterkin has difficulties with shots from specific angles, targeted drills can be developed to enhance his skills in those areas.
Optimizing Scoring Capabilities: Andrei Svechnikov
Similarly, machine learning analyses of Carolina Hurricane’s Andrei Svechnikov focus on shot conversion rates, positioning, and puck handling under pressure. Insights derived from this data help Svechnikov and his coaches understand his performance peaks and troughs, allowing for focused improvements in scoring strategies and in-game decision-making.
Bridging the Gap Between Data and Training
The integration of machine learning provides a bridge from theoretical data to practical application in training regimes for teams like the Carolina Hurricanes and New York Rangers. This approach involves not just individual player analysis but also strategic adaptations that enhance overall team performance.
Impact on Team Strategies
For teams like the Rangers and Hurricanes, machine learning offers a strategic overview that informs game plans and opposition analysis. This technology allows teams to exploit weaknesses in opponents while shoring up their own areas of vulnerability, leading to more informed and strategic gameplay.
Challenges and Ethical Considerations
Despite its benefits, the use of machine learning in NHL analytics is not without challenges. Concerns about data privacy, the potential for misinterpretation of data, and the ethical use of AI in sports remain prevalent. Balancing technology with traditional coaching methods and ensuring fair play continues to be a priority for the league.
Conclusion
The use of machine learning to enhance the abilities of players like Igor Shesterkin and Andrei Svechnikov exemplifies the cutting-edge of sports science in the NHL. As teams like the New York Rangers and Carolina Hurricanes continue to implement these advanced analytics, they not only improve individual player performances but also reshape strategies at a team level. The future of NHL performance analysis is here, with machine learning leading the charge in transforming how teams approach
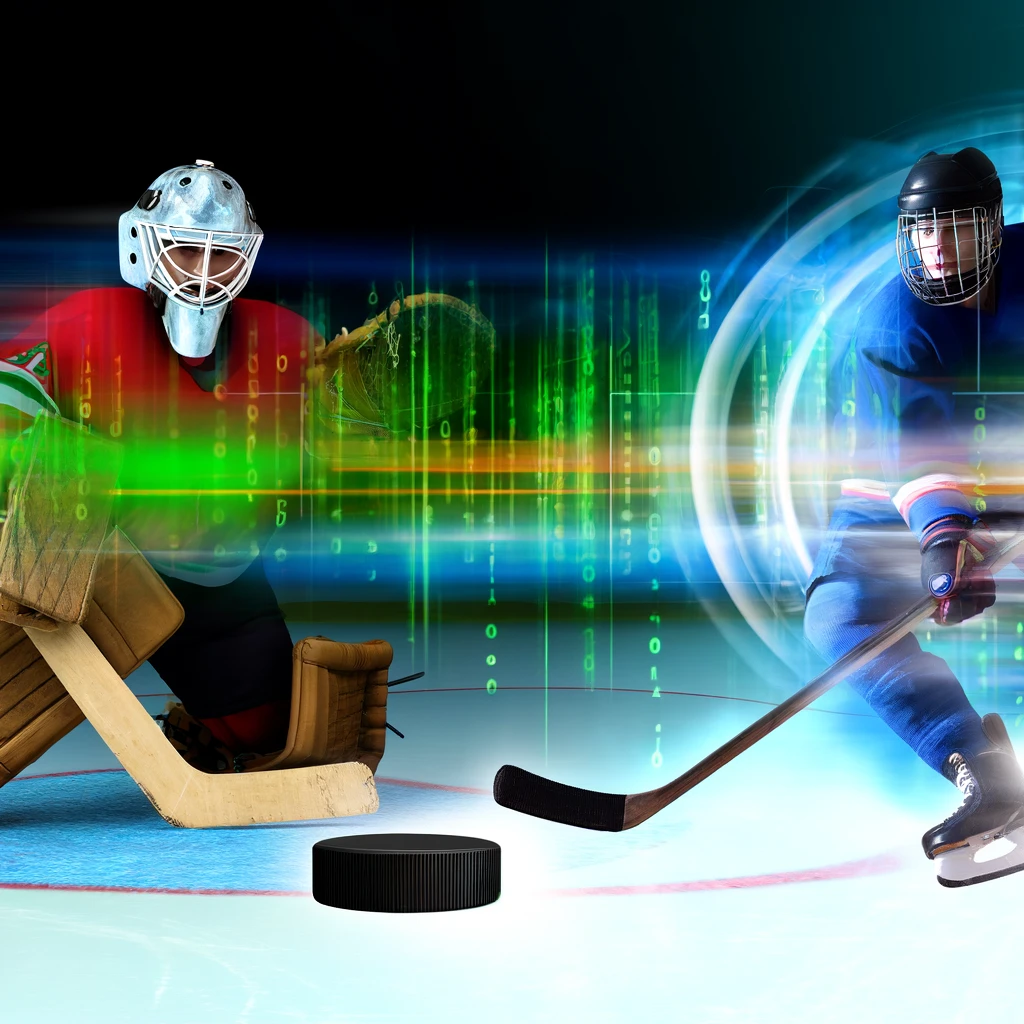